Case Study
Scaling an Online Pharmacy Using AI and Automation for Insurance Verification
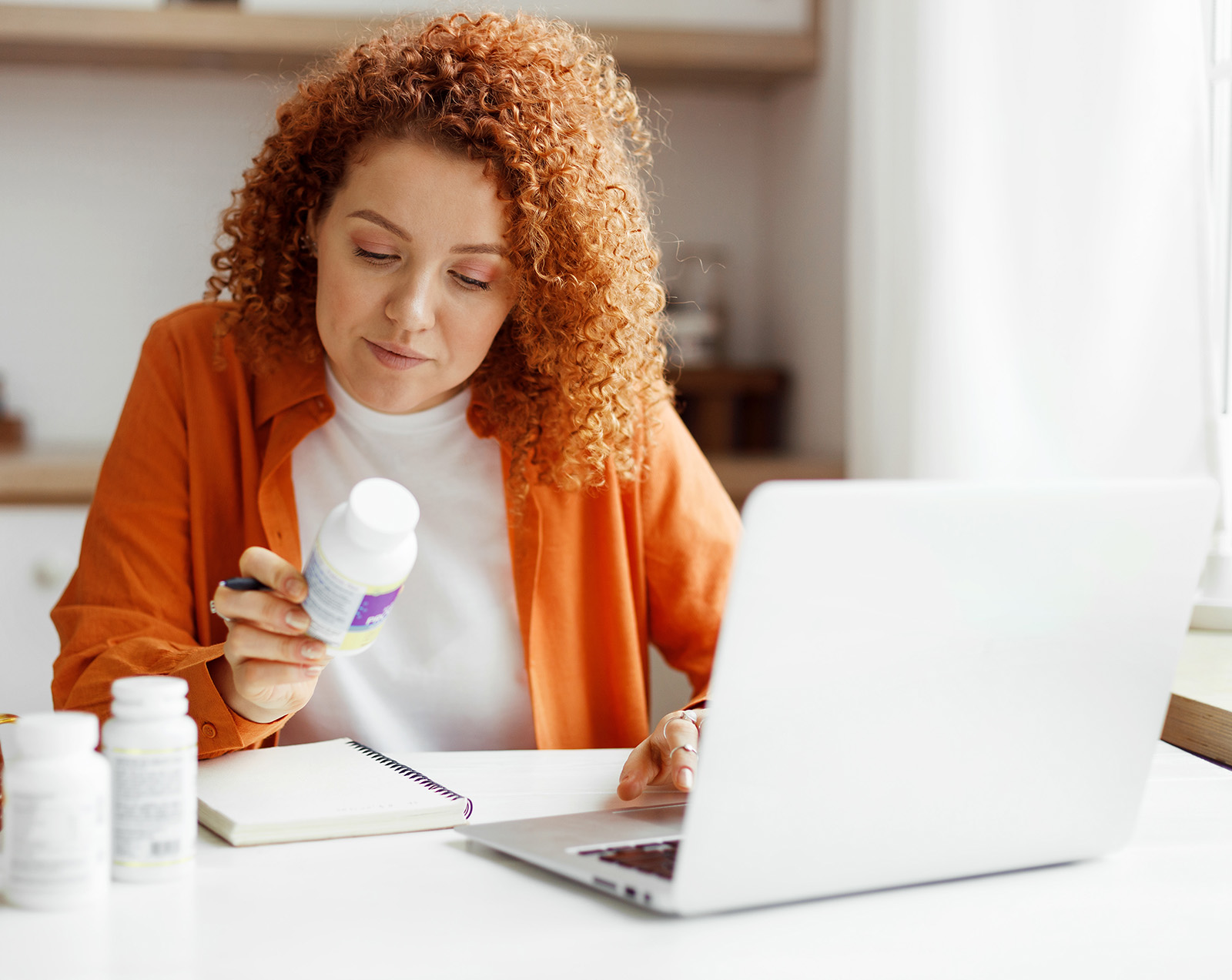
TABLE OF CONTENTS
The Customer
A U.S. pharmaceutical company approached the Roots' team with interest in an AI Agent for verifying medical ID cards. This $600 million dollar company was growing by 200% month-over-month, and their 200 employees were at capacity.
This organization was breaking into the healthcare industry by providing easy-access to contraceptive care for women, specifically for birth control pills. The company wanted to optimize their new user experience by implementing automation within their medical insurance verification process. The goal of the partnership centered around 1) decreasing the time that the team was spending manually verifying insurance information via medical ID cards, and 2) decreasing the wait-time before the user could use the service.
Alternatively, the operations executives would have to triple the size of their insurance eligibility verification team to keep up with demand and to meet the users’ expectations on speed.
The Situation
This Customer provides contraceptives and other forms of healthcare to their growing subscriber base. Each month, this rapidly expanding company grows by 20,000 new subscribers. At the beginning of our partnership, this translated to roughly a 200% month-over-month increase in users.
Because this Customer accepts different forms of medical insurance, they needed to verify insurance eligibility as customers sign up. Additionally, the insurance eligibility would need to be re-verified every month thereafter. The Customer employed 7 people offshore and 2 people onshore to read, extract, and verify insurance information for each subscriber, but both teams were quickly overwhelmed by the company’s growth.
The teams were manually reviewing uploaded pictures of medical insurance ID cards, extracting the data and entering the information either into the company’s systems or into insurance company websites to validate coverage and eligibility.
The Challenge
The Customer’s accuracy level on manual insurance eligibility verification was low, at a 70% rate of accurately extracting and verifying insurance. The Customer’s subscribers were forced to wait at least 24 hours while the company validated insurance coverage, and then the company needed 5 business days to ship their product to their user. This unfortunately created a poor experience for new users and existing users alike – especially because the company provides contraception prescriptions, which can be a time-sensitive need for their subscribers.
Meanwhile, the company’s growth of 20,000 new subscribers each month was increasing the workload and the Customer could not scale effectively while simultaneously increasing their payroll. With the increased workload, the Customer was at a critical point as an organization.
The Solution
Every insurance card is different, so we needed to train an AI Agent to recognize the information despite the unstructured format. Every insurance company has its own insurance card, and employers may have multiple versions of their insurance cards. Because of this variation, a traditional RPA exercise wouldn’t have been successful because the data would always be unstructured and in an unpredictable format. Additionally, subscribers submit their own photos of their medical ID cards, occasionally resulting in a skewed or dithered primary source.
Roots team used Machine Learning, Natural Language Processing (NLP), Intelligent Document Processing (IDP), and Computer Vision to recognize the subscribers’ submitted photos of insurance cards. The Digital Coworker reads the picture of the medical ID card using OCR, extracts the data, and then passes the data to the NLP engine for classification. Then, an API is used to verify if the subscriber is actively insured. Accuracy levels quickly rose from 70% (via manual output) to 95%. A process that once took 24 hours now takes less than 1 minute. This Customer experienced a 900% ROI.
In today’s process, the ask of the subscriber has not changed; the Customer requests that users submit a photo of the medical insurance ID card for verification. Then, using sophisticated Computer Vision algorithms, the image is then D-skewed and the image quality is improved. Then, the AI Agent uses OCR to read all the data on the card before passing the information over to the NLP engine, at which point the AI Agent is able to read the card number, the subscriber, the primary insured, the insurance and pharmacy information, the employer of the subscriber, and their membership ID. Nothing in this process is templatized.
After the Machine Learning engine takes over, the AI Agent will determine whether it is confident in the information it has extracted. If the answer is yes, the AI Agent will initiate the API into the Customer’s systems to verify whether this person is insured or not, and then returns the results. If the API can’t verify the information in the Customer’s systems, then the AI Agent will navigate to the website of the appropriate insurance carrier, enter the patient’s details, read the results, and bring said results back to the Customer’s system.
Ultimately, this was an incredibly successful partnership, with a 900% return on investment for our Customer.
The team of 9 was reduced to only 2 FTE, who now work alongside the AI Agent in case it has any questions. The remaining onshore employees were upskilled and reskilled into different areas of the growing operation. Additionally, the accuracy of the extracted information rose from 70% to 95%, improving the customer experience by reducing wait times due to manual error.
The Customer saw a significant improvement in throughput, as the AI Agent transformed a 24-hour turnaround into a process that takes less than 1 minute, from start to finish. Whereas the Customer originally needed 24 hours to verify insurance information and another 5 business days to ship the product to the subscriber, the AI Agent enabled our Customer to reduce this timeframe to 48 hours. This significantly improved the user experience.
Thanks to the AI Agent, this Customer was able to improve their overall operations and present these improvements to their board of directors. Eventually, this organization went on to expand their focus to the wellness of all genders, though it still centers around contraceptives.